Before Pareto, franchisees were left guessing, relying on unactionable data and heuristics
For franchisees, corporate provided competitor pricing reports showing what nearby chains were charging, along with rough guidelines on market conditions. But those excel files were difficult to digest and still didn’t answer the real questions:
1️⃣ What’s the right price for each SKU in my market?
2️⃣ If I change the price of SKU X, how will that impact sales of SKU Y?
3️⃣ What set of prices will allow me to maximize my total profits in the near and long term?
In addition to the limited data provided by corporate, franchisees relied on heuristics such as pricing such that production costs are always 30% of menu costs which is an old industry adage seemingly based on zero data.
Seriously, that is how things were done before Pareto: raw excel sheets and adages. For the most part, franchisees knew that with these methods, they were all but guessing at the answers to these questions, but they were ultimately making the best decisions given their limited resources.
Corporate knew franchisees needed data to make informed decisions, but this was easier said than done
While franchisees ultimately control their own pricing, corporate recognized the power of helping them get it right. They did what they could—equipping stores with raw data to inform pricing decisions:
✅ How many competitors were within 1, 5, and 15 miles.
✅ What those competitors charged for benchmark products like burgers and drinks.
But this data wasn’t actionable. Franchisees—already busy running their stores—couldn’t easily translate competitor pricing spreadsheets into an optimized pricing strategy.
Corporate’s attempt at a smarter internal pricing framework
Recognizing these challenges, corporate attempted to develop an internal pricing framework to reduce franchisee guesswork. While they lacked the machine learning (ML) resources of McDonald’s or Starbucks—who have spent over $1 billion developing in-house pricing optimization tools— they did the best they could under their constraints.
Their approach? Use competitor pricing to estimate local pricing power.
➡️ If McDonald’s charged $4.79 for a Big Mac while the market average was $5.99, corporate would recommend franchisees in that area price below the national average across the board.
➡️ Conversely, if local competitors priced higher than average, they encouraged franchisees to charge more.
This was an early recognition that data-driven pricing was the future. It was, in essence, an attempt to replicate what McDonald’s had built internally—but without the benefit of advanced ML models to dynamically adjust for SKU interactions, demand elasticity, and differing customer preferences between brands.
What was missing? Why even this wasn’t enough.
Despite the revenue team’s best efforts, their solution ultimately boiled down to a sophisticated version of a follow-the-leader approach to pricing which firms have been using for hundreds of years to set prices. While copying competitors is intuitive, the necessary assumptions are far reaching and prove false.
❌ Assumes demand between across brands is identical ➡️ if there is strong demand for McDonald’s in this area, then there must similarly be strong demand for our stores as well.
❌ Assumes competitors are themselves always pricing optimally ➡️ If McDonald’s prices above the national average then that must be the optimal price.
❌ Assumes that demand between SKUs is constant ➡️ If there is low demand for sandwiches in this area, there must similarly be low demand for coffee.
❌ Assumes there are no inter-SKU relationships ➡️ If we increase the price of fries by 5%, there will be no impact on sales of fountain drinks.
While their intuition was spot on and was an improvement over the raw spreadsheets of competitor data, it failed to drive meaningful change as the necessary assumptions do not hold true.
A shared vision for the future
With franchisees demanding data-driven pricing recommendations and the corporate revenue team seeking an advanced solution to meet this need while maintaining oversight, deploying Pareto Pro emerged as the optimal choice.
Pareto Pro provides franchisees with monthly SKU-level pricing recommendations via an intuitive online interface, enabling them to assess product relationships—identifying which SKUs compete and which complement each other. While franchisees retain final pricing authority, their decisions are now informed by rigorous econometric modeling rather than intuition or the manual analysis of endless Excel files.
For the corporate revenue team, Pareto Pro eliminates the inefficiencies of distributing and managing tens of thousands of ad hoc Excel files. Instead of diverting resources to building internal tools, the team can now focus on high-impact strategic initiatives, confident that franchisees are operating with the best possible data. The platform’s Brand Control Dashboard further ensures alignment with corporate strategy by maintaining relative price relationships, setting guardrails on month-over-month price movements, and allowing the revenue team to approve recommendations before they reach stores.
What Pareto did differently
Pareto is unlike any pricing tool before it—it reimagines pricing optimization by combining advanced econometrics with machine learning while keeping franchise and corporate teams in the driver's seat.
What Pareto did not do:
❌ Make pricing dynamic by the hour—constant price changes frustrate customers and weaken brand loyalty.
❌ Eliminate human expertise—revenue teams offer invaluable insights and remain central to the decision-making process.
❌ Rely on industry folklore or gut instinct—We leave folklore to poets and let mathematicians handle optimization.
What Pareto did do:
✅ Leverage proven econometric principles used by leading big tech firms to forecast demand and set optimal prices.
✅ Harness the latest in AI and machine learning to make cutting-edge pricing technology accessible to brands of all sizes.
✅ Train on thousands of variables, from the obvious—median income—to the overlooked—which side of the road a store is on.
Pareto democratized access to cutting-edge pricing technology, bringing advanced econometric modeling and machine learning to brands of all sizes. Franchisees gained the actionable insights they had always wanted—delivered in a clear, easy-to-use format that required no additional time or analysis on their end. Meanwhile, the corporate revenue team unlocked a new level of pricing intelligence, enabling them to provide the depth of analysis that was previously out of reach. With Pareto, they could not only deliver smarter recommendations but also guide pricing strategies through their strategic oversight, ensuring alignment with broader business objectives.
What happened next? The results were game-changing
The financial impact was undeniable. Stores that implemented Pareto’s recommendations saw an average 15% increase in revenue and an 80% boost in net profit, proving the power of data-driven pricing.
Beyond the numbers, the operational impact was just as transformative. Franchisees no longer had to second-guess pricing or spend hours buried in spreadsheets. Instead, they could focus on running their stores, delivering a great customer experience, and driving demand. Meanwhile, the corporate revenue team was freed from manually cleaning and distributing raw data. With Pareto handling the econometrics, they could instead dedicate their time to high-impact strategic initiatives—optimizing promotions, fine-tuning brand strategy, and ensuring every location had the tools to maximize profitability.
Franchisees and revenue teams always knew pricing could be better. McDonalds and Starbucks spent billions building in-house solutions; Pareto makes that power available to everyone else.
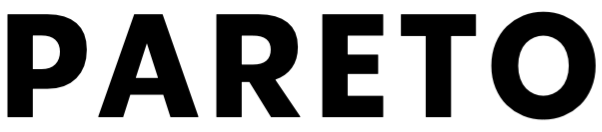
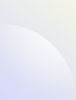